In this project, Michelle Lee and Todd Presner explore tens of thousands of questions asked to survivors during the foundational years of four Holocaust archives:
- • David Boder’s interviews: Topical Autobiographies and Voices of the Holocaust (1946)
- • Yale Fortunoff Video Archive for Holocaust Testimonies (1979-1983)
- • USC Shoah Foundation’s Visual History Archive (1994-1996)
- • USC Shoah Foundation’s Dimensions in Testimony (2018-2021)
We critically assess how machine learning language models can help us classify and understand the changing topics and questions asked to survivors over seven decades, across four testimonial corpora. The outputs are a series of interactive visualizations that allow readers to explore 89,759 questions and trace changes over time. The questions can be explored all at once, by testimonial corpus, or by individual testimony, the last of which allows users to compare the topics covered by an interview in the order in which they were asked. As we argue in the chapter, the machine learning processes are not automatic but rest upon a series of decisions and assumptions at different stages in the process. We discuss how the outputs should be considered as “subjunctive metadata” — that is, possible and plausible outputs given the constraints we assigned, the interpretative decisions we made, and our understanding of the operations of the algorithms (SBERT and k-means clustering). Such an approach highlights the importance of the humanities in interrogating the presumed definitiveness of algorithmic approaches for analyzing the cultural record.
Tableau visualizations are also available on our public Tableau page.
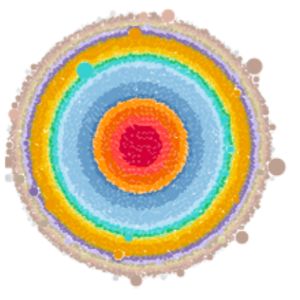
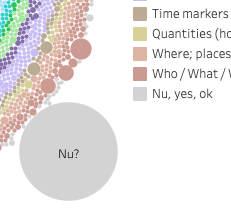
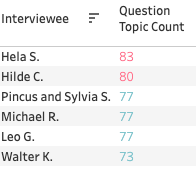
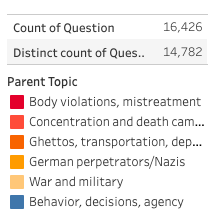
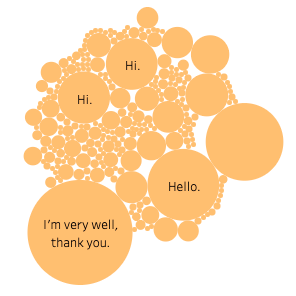