About us
Started in Summer 2024, the AI and Cultural Heritage Lab is an extension of the Digital Humanities Holocaust Research Lab. In partnership with various digital libraries and archives, the AI Lab is investigating how Large Language Models (LLMs) can be used to enhance metadata, develop new finding aids and indexing systems, and deepen our analysis of complex cultural data.
Our team works with a wide range of data including genocide testimony transcripts, AI-generated answers to Holocaust queries, criminal court records, Google search data, and encyclopedia entries about the Holocaust and other genocides.
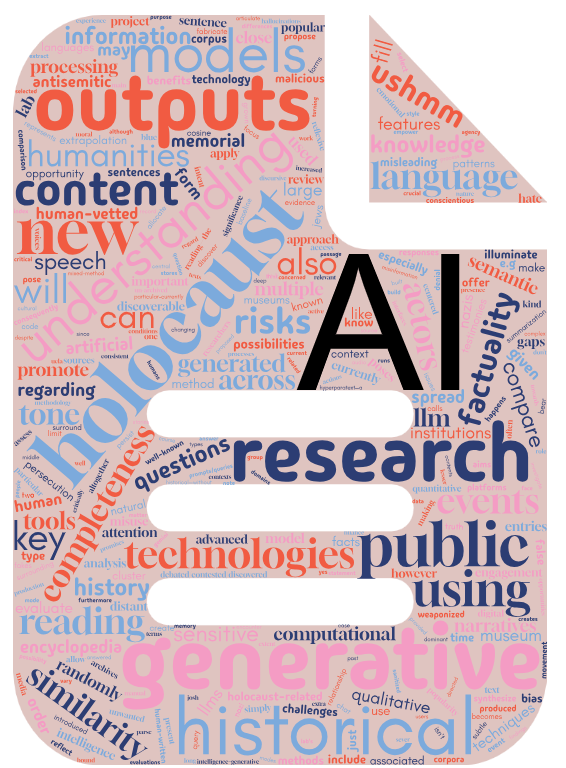
Research areas
• Cultural memory – using AI to reconstruct and communicate historical memory.
• Large reading – a scalable, repeatable practice of interpretation in which language models are used to identify, render, and synthesize qualitative distinctions across massive text corpora.
• Rich content indexing – using AI to help create richer content indexes capable to surface narratives, emotions, and tone nuances in large textual and video archives.
• Knowledge graphs – using LLMs to disambiguate data and build robust knowledge graphs to explore identities and relationships in dynamic environments.
• Metadata generation – using LLMs to generate accurate and reliable metadata for digital collections.
• Deep contextualization – using LLMs to help create deep contexts for understanding the content of documents.
• Computational Exegesis – applying generative AI to surface latent structure, variation, or discursive logic in testimonies, borrowing from hermeneutics and literary theory.
• AI influence on historical memory – examining the role of AI-based tools and AI-generated content on users’ perception and learning of Holocaust-related and, more broadly, contested historical events.
Lab projects
AI for content disambiguation
We use AI to contextualize and disambiguate statements, pronouns, and implicit references mentioned by survivors in Holocaust testimony transcripts (for example, when a survivor says “he saw that“, who is “he”? What is “that”?) This helps us implement further analysis methods like question clustering or topic modeling that can take into account a fuller picture of the witness’ narrated experience.
Question clustering
We explore various AI-based methods to cluster the questions posed to Holocaust survivors and categorize them into different themes, building on our previous question analysis work that analyzed the way interviewers interact with survivors in three audio and audio/video archives of Holocaust survivor testimonies.
Kaleidoscopic Indexing
When searching an archive, indexes help us find content and suggest categories of content worth looking for. But what is “content”? We use AI to support deeper modes of historical engagement with survivor testimonies: we develop humanistic heuristics to direct AI to index testimonies not only for factual content but also for tone, agency, narrative stance, silence, and moral position.
LLMs and Holocaust research
In partnership with the US Holocaust Memorial Museum, we analyze the top Google queries related to the Holocaust that led Internet users to the USHMM encyclopedia, and compare the answers provided by USHMM encyclopedia entries and other forms of human-generated content provided by Google with AI-generated answers provided by various Large Language Models (GPT, Gemini, Grok, DeepSeek) as well as Google’s AI Summary. We compare how they perform in terms of factuality, but we also explore how users can be exposed to hard to pinpoint differences like tone, completeness, biases, positionality, and moral or educational stances.
Knowledge Graph-based Indexing
We explore non-linear ways of reading and understanding survivor interviews by graphing the people, events, and objects they mention. Building upon our previous work on semantic triplets, we use AI to identify and label nuanced relationships connecting the subjects mentioned in Holocaust survivor interview transcripts.